What is Retrieval-Augmented Generation (and why should every legal professional know about it)?
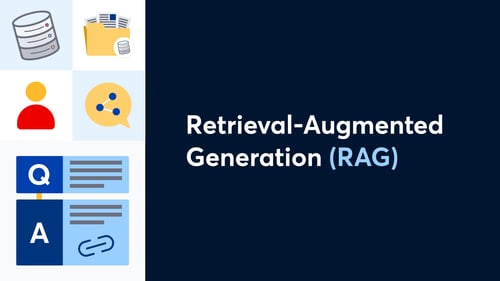
Generative AI (GenAI) has quickly emerged as a top investment priority for many companies, a trend underscored by the recent EY Reimagining Industry Futures Study. This study showed that 43% of the 1,405 enterprises surveyed are investing in GenAI technology. Another study from Infosys estimates a 67% increase in company investments in GenAI over the next year. However, while excitement about GenAI remains high, so does the uncertainty surrounding it.
A global study by MIT Technology Review Insights revealed that as many as 77% of participants see regulation, compliance, and data privacy as significant hurdles to the swift adoption of GenAI. Legal teams investing in GenAI face additional challenges, such as a lack of specialized knowledge, mistrust, and fear of producing "hallucinations" — inaccurate or misleading information.
However, the outlook for GenAI is not all doom and gloom.
A viable strategy for overcoming these obstacles is to augment Large Language Models (LLMs) with unique, proprietary data. This approach not only improves the accuracy, relevance, and overall integrity of the outputs but also mitigates many concerns related to regulation, compliance, and privacy.
In this blog, we'll explore how Retrieval-Augmented Generation (RAG) improves the quality of LLM predictions and provides benefits to legal teams considering the use of GenAI applications.
First, let’s start with the basics: What exactly is RAG?
What is Retrieval-Augmented Generation (RAG)?
Retrieval-Augmented Generation (RAG) is a natural language processing technique used to improve LLM prediction quality. In a RAG workflow, LLMs reference domain-specific datasets to inform their responses, yielding more relevant answers. RAG workflows can reduce hallucinations and produce contextually relevant responses. This utility makes RAG particularly useful for tasks requiring up-to-date information or in-depth knowledge of specific topics.
In the context of legal applications, RAG workflows can produce responses that are grounded in actual legal principles and precedents. This capability makes RAG especially useful for summarizing internal knowledge, drafting new legal documents, and providing advice based on legal precedent.
How does Retrieval-Augmented Generation work?
RAG combines the power of LLMs with external datasets to produce highly accurate and contextually relevant text outputs. Here’s a step-by-step breakdown of how RAG works:
- Dataset curation: As a first step, it’s critical to define the curated dataset that the LLM will reference. This involves centralizing, cleaning, auditing, and preparing the data for use by the LLM.
- Calculate vector embeddings: Calculating vector embeddings is a key part of preparing data for a RAG workflow. Vector embeddings are numerical representations of text designed to capture the semantic relationships between words. These embeddings are essential for powering the retrieval process, as they facilitate the identification and retrieval of relevant information from the dataset (more on that in step 5).
- Store vector embeddings: After calculation, vector embeddings are stored in a vector database to facilitate quick retrieval.
- Query submission: A RAG workflow begins when a person submits a query to an LLM-powered application, such as a chatbot or a search UI. For example, a user might ask a chatbot, "Can you summarize the changes to our internal compliance policies from the last six months?" The application analyzes the query and calculates its vector embeddings.
- Information retrieval: The vector embeddings from step 4 are used to "search" through the external dataset configured in step 1. A matching process occurs between the vector embeddings calculated in steps 2 and 4. The vector database returns the stored documents that best match the query text, based on semantic similarity.
- Relevance scoring: Some RAG workflows then use relevance scoring to improve retrieval accuracy by ranking and ordering the retrieved information.
- Hybrid search retrieval: In addition to using vector embeddings to find relevant documents, some RAG workflows also employ keyword retrieval mechanisms. This hybrid approach further improves retrieval accuracy.
- Response generation: The retrieved documents are used to create a prompt that is sent to the LLM. This prompt incorporates both the user's query and the retrieved content to generate and deliver a contextually relevant response to the user.
- Output refinement: Before reaching the user, the generated response might undergo refinement to ensure coherence, relevance, and factual accuracy.
- Response: The response is delivered to the user, sometimes including reference links. One of the benefits of RAG is its reference capability. It’s possible to include reference links to the sources used to inform the response. This information can be used to further improve the workflow.
How does RAG compare to fine-tuning?
Another popular method for incorporating proprietary and domain-specific data into LLMs is fine-tuning. In this process, a pre-trained model is further trained (or "fine-tuned") on a smaller, specific dataset. Fine-tuning allows the model to adapt its pre-existing knowledge to better suit the nuances and specifics of a particular domain or set of proprietary data.
In legal applications, fine-tuning a model on legal texts, case law, and legal literature helps it grasp the nuances of legal language, terminology, and concepts. This enhanced understanding enables the model to perform complex tasks more effectively, such as analyzing contracts, interpreting statutes, or predicting legal outcomes with a higher degree of precision.
Both RAG and fine-tuning augment AI model capabilities in specialized areas and can be used either separately or in combination, depending on specific needs and objectives. While RAG enhances the LLM’s ability to retrieve and utilize existing information, fine-tuning tailors the model's core understanding and processing abilities to domain-specific language and concepts. When used together, these methods synergize to equip AI applications with the ability to generate highly accurate, relevant, and contextually aware outputs.
Overcoming legal considerations with RAG: Enhancing security, efficiency, and ethics
RAG, in particular, is gaining popularity for its efficiency in integrating proprietary data sources in a way that’s secure, transparent, and cost-effective. Here’s how RAG can address common considerations posed by legal teams:
Data privacy concerns
RAG addresses data privacy concerns by enabling legal professionals to securely leverage their own internal data repositories. Instead of relying on public datasets, RAG facilitates the retrieval of relevant information from proprietary sources. This approach ensures that sensitive information remains within the organization's control, reducing the risk of data breaches or exposing sensitive data to unauthorized users. Through fine-tuning, organizations can curate and vet the data sources utilized by RAG, ensuring that the AI-generated content aligns with data privacy standards.
Ethical considerations
RAG helps address ethical considerations by providing transparency and control over the information used in AI-generated outputs. Its ability to retrieve contextually relevant information improves the accuracy of both generic and fine-tuned foundation models. Ideally, the RAG workflow includes links to the retrieved context within the LLM response, allowing users to validate the responses. This approach serves as a means to combat bias and protect against hallucinations.
RAG also enables data lineage for LLMs by offering the ability to trace the origin and history of the data used in AI-generated outputs. By retrieving information from specific sources within proprietary data repositories, RAG provides transparency and accountability, ensuring that AI-generated content is grounded in verifiable and trustworthy data sources.
Onna has created an internal Slackbot using a RAG workflow, which enables team members to search for internal information and provides reference links.
Cost and resource constraints
RAG offers a cost-effective solution for implementing LLMs by leveraging existing proprietary data sources. Utilizing internal data repositories enhances the reliability of the generated content by ensuring it is directly linked to verifiable internal data. This not only makes the content more credible but also minimizes the risk of generating inaccurate information.
Additionally, RAG can streamline the process of finding and producing legal content, automating what are typically manual and time-consuming tasks. This efficiency saves significant time and effort that is usually spent on manually finding and inputting information for use with LLMs.
Looking forward: Preparing your data for AI
It's clear that the buzz around LLMs in legal tech is not just hype — it's a signpost toward the future. Techniques like RAG and fine-tuning are effective strategies for creating more reliable and successful AI applications, ensuring that your proprietary data doesn't just sit there but actively works for your business.
If your organization is among the 70 percent investing time, resources, and money in GenAI, or if it is considering doing so, you shouldn't overlook the importance of building a comprehensive data infrastructure. A well-prepared data foundation ensures that AI models like LLMs have the high-quality, domain-specific data they need to generate accurate and contextually relevant results. Leveraging your proprietary data with LLMs will unlock opportunities to successfully use Generative AI across your organization.
If you’d like to dive deeper into RAG and understand how it can be used to build effective AI applications, check out our AI Data Pipeline solution or get in touch here.