Responsible adoption of generative AI: A four-step framework for organizations
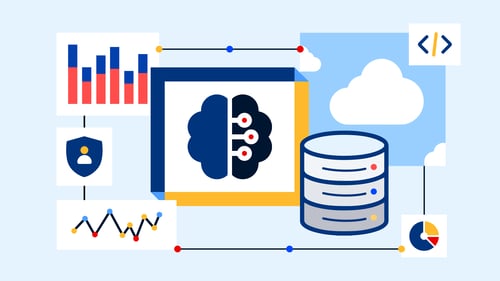
Maximizing the value of AI and Large Language Models (LLMs) begins with developing a strategy and a secure workflow to incorporate proprietary data for enterprise use.
But how can organizations ensure that these technologies are ethically and responsibly adopted — and utilized?
In a recent EDRM webinar, Balancing Offensive and Defensive Governance Strategies for Generative AI, our CEO, Kelly Griswold, joined Michele Goetz, Vice President and Principal Analyst with Forrester, and Travis Bricker, Senior Program Manager, Legal, at Coinbase, to answer this question. They discussed four steps that will help organizations move beyond wishful thinking and hypothetical applications of generative AI to discover practical applications that adhere to data governance principles and protect proprietary and sensitive data.
Rather watch than read? Check out the full webinar on-demand now.
Step 1: Define the problem(s) you want to solve
There are numerous use cases for generative AI, from generating and analyzing contracts to drafting pleadings and motions, reviewing evidence for eDiscovery, and managing knowledge stores. So, where should an organization, or more likely, teams within an organization, begin?
The panel recommended that interested parties start by understanding the challenges you want to solve. AI is particularly useful for repetitive, time-intensive processes, such as information retrieval and summarization.
Bricker explained that Coinbase found use cases for generative AI by thinking about the problems the legal team was facing, such as summarizing subpoenas. He asked an LLM to review dummy subpoena data, summarize it, and route the subpoena appropriately. The tool provided correct answers, demonstrating that that was a use case the LLM could solve.
Next, he tried using the LLM to streamline knowledge management tasks, such as summarizing legal memos, opinions, and internal and external documents. The team could then ask the LLM to answer questions about the documents based on the collated information. He observed:
“The problems we wanted to solve didn’t come from thinking about what AI can do but about the problems we have.”
To identify potential use cases for generative AI, consider questions like these:
- What repetitive tasks do you spend significant time on?
- What tasks do you frequently get stuck on?
- Which parts of your daily work routine frustrate you or consume too much of your time?
- Where is there friction in your current workflows or processes?
- If you had an assistant or intern available at all times, what tasks would you assign to them?
Griswold advised that organizations should understand the user stories behind the use cases. “Talk about the challenge and the outcome. If you look at practical business problems with that lens, it will accelerate adoption.”
Once you have identified the use cases, use a common framework, such as a prioritization matrix, to help you decide which cases to prioritize, which to defer to later, and which to discard.
Currently, LLMs may not be suitable for certain types of tasks, such as those that primarily rely on interpreting non-textual data or those that heavily depend on cultural, regional, or contextual nuances. In these cases, other solutions might be more effective. Two important considerations to keep in mind are:
- LLMs are more than what you need for simple tasks. While they can perform well, they are also expensive. If a simpler technical solution can perform just as effectively as the LLM, it will likely be more cost-effective.
- For highly specialized or high-risk tasks, optimization will require more effort, and your experts or humans may need to remain more involved in the process. Consider not tackling these tasks first — build your internal expertise and let the technology mature before taking them on.
Step 2: Understand the risks of using generative AI (and plan accordingly)
The panel agreed that organizations interested in using their proprietary data with LLMs and generative AI would be wise to start by experimenting with low-risk use cases. Top concerns about generative AI include privacy and data protection, with 61% expressing apprehension in a recent Forrester survey, according to Goetz. Misuse of AI outputs (57%) and the potential for employees to use unsanctioned AI (52%) were also significant areas of concern.
The key is to determine whether an incorrect response from the technology would cause harm. For example, if you use generative AI with a dataset that contains internal documents drafted by in-house counsel, would you lose the protection of attorney-client privilege?
Bricker advised organizations to limit the magnitude of any errors by keeping the initial use cases internal only. To further mitigate risk, organizations should check the security measures and permissioning controls of any tool that enables connecting proprietary data to an LLM. Permissioning controls ensure that highly sensitive data from enterprise applications will not be accessible to LLMs.
Step 3: Determine a cost-benefit analysis between building or buying a generative AI solution
The panel then discussed the merits of building versus buying a generative AI solution. Bricker recommended revisiting user stories, evaluating your budget, and determining your timeline to decide on an implementation approach. Many companies are launching AI features and tools that can enable small but important wins, and help build familiarity with AI in the organization. “You don’t always have to implement a tool across the company. You may just need to buy or turn on a particular feature. Small features can help you solve the problems your users come up with.”
Building a generative AI solution gives organizations greater transparency into how their technology is functioning, as well as better control over their data. On the other hand, constructing a generative AI solution can be a lengthy process that may take anywhere from 6 to 18 months to complete. Additionally, it necessitates consideration of the level of internal expertise required.
However, Griswold elaborated on various techniques that can be used to streamline the development process, such as Retrieval Augmented Generation. The costs of building a proprietary solution are also likely to even out over the long term, and the decision to build or buy ultimately depends on your use cases and priorities.
Consider the following questions when deciding whether to build or buy:
- How can I best leverage my existing vendor relationships? What are my current vendors offering or doing in this space?
- For successful POCs: What steps are needed to scale up? How will the costs and benefits appear at a larger scale?
- For unsuccessful POCs: Wind it down. What lessons can we learn from this?
- "Moving to Production": Who is responsible for maintaining the solution? What is the protocol when something breaks — who will address it? Assess the Total Cost of Ownership (TCO) over a specified period, including overhead, maintenance, and support.
It's also important to consider the composition of your team. Do you have the in-house expertise to build a solution, or would you be better served by a vendor's existing technology? Evaluate your team's strengths and limitations to make an informed decision. Depending on who is part of your team, and their specific skills and experience, you may find it more effective to approach step 4 before step 3, or vice versa.
For instance, if you have a strong technical team, you might prioritize building your team (step 4) to leverage their skills in the decision-making process of whether to build or buy (step 3). Conversely, if your team lacks in-house technical expertise, it's advisable to seek guidance from a technical expert to conduct a comprehensive cost-benefit analysis (step 3). This analysis will help you understand whether acquiring external resources is necessary before assembling a dedicated team (step 4).
Step 4: Form your team
Once an organization has defined a use case, assessed the risks, and chosen a solution, it needs to assemble a team with three critical roles: the business user, the technical expert, and the prompter/tester.
The business user defines the use case. Their role is to outline — in plain language — the job requirements, workflows, challenges, and desired outcomes.
The technical expert is responsible for building, deploying, and operating the model. If you don’t have someone in-house who can fill this role, a vendor or other technology partner can step in, particularly if they are integrating this feature into their existing software.
Finally, the prompter/tester ties it all together, translating the business user’s needs, actions, and output into effective prompts for the model. They then test and refine those prompts for accuracy.
These three roles can help move an organization out of the think tank stage and into finding practical applications for generative AI that add value to the business without creating undue risk.
Start exploring the applications of generative AI
As organizations navigate the evolving use cases of generative AI with their proprietary data, they must determine how to use these models without running afoul of data governance principles. These four steps offer a practical roadmap to help organizations unlock the potential of generative AI responsibly.
Check out the on-demand webinar to learn more about using generative AI responsibly in your organization, or reach out to us to discover how Onna can assist you in connecting your proprietary data to generative AI solutions.