3 practical applications of Retrieval-Augmented Generation for legal teams
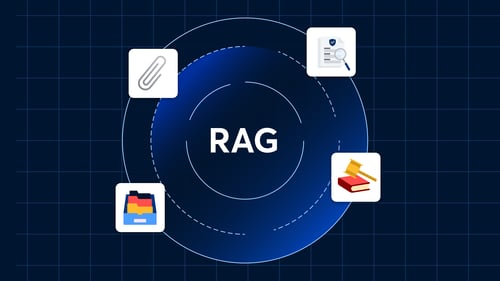
Legal professionals are no strangers to performance and productivity-related tools. From matter management to legal workflow tools, these solutions allow them to do their jobs better and with greater accuracy.
Now, with the rising adoption of Generative AI (GenAI) technology, many corporate legal departments are leaning into AI to deliver smarter and more efficient legal work.
In a previous blog post, we explored how Retrieval-Augmented Generation (RAG) improves the quality of large language model (LLM) predictions and highlighted the benefits it offers to legal teams considering the use of GenAI applications.
In this blog, we’ll go a step deeper and explore a selection of use cases where RAG's retrieval capabilities and content generation functionalities are most impactful, especially when used with proprietary data. From summarization and review to discovery process optimization, here are 3 examples that demonstrate how RAG’s ability to retrieve, summarize, and reference documents can improve legal workflows.
Automated summarization and review
For corporate legal teams, having the ability to quickly search, retrieve, and summarize internal and outside counsel advice is highly important, as it allows them to leverage previous work and guidance. This proves especially valuable for larger companies that may be managing multiple cases at once and need to honor historical precedents. With GenAI, a company can build a search UI or a chatbot that uses a repository of former depositions and legal decisions to provide responses. Legal teams can then use the GenAI application to prepare for litigation and any other legal situation facing the company.
RAG can retrieve relevant information from extensive content repositories and generate concise summaries, making it well-suited for this use case. This capability is crucial for quickly distilling past legal advice, case precedents, and testimonies into actionable insights.
Example: Legal advice
Scenario: A law practice specializes in product liability matters on behalf of Company ABC. Over the last 15 years, Company ABC has been involved in similar litigation and has engaged the services of the same expert, John Doe, on several occasions. Testimony has been provided in the form of both transcript testimony and summaries from outside counsel to Company ABC. Outside counsel and Company ABC are exploring the possibility of engaging expert John Doe for a new matter.
Discovery process optimization
RAG can also optimize the eDiscovery process by automating tasks such as document retrieval, summarization, and analysis. As mentioned above, RAG can quickly retrieve relevant documents and generate summaries or key insights, reducing the time and resources required for eDiscovery.
Example: Similar incident lawsuits
Scenario: A medical manufacturer is working with new outside counsel on litigation for a specific device. As of the end of last month, 16 matters have been litigated for this device. The company is trying to ensure that it can provide the outside counsel with the advice from the law firms they last worked with.
Compliance audits
RAG can enhance the efficiency of verifying an organization's adherence to legal standards, making it a useful tool in internal compliance audits. By automating the retrieval and analysis of legal documents, it alleviates the often tedious task of locating and sifting through information.
Example: Employment law compliance
Scenario: The HR department is revising company policies to ensure compliance with new employment laws and needs to consult both internal legal advice and opinions from outside counsel to align their policies accordingly.
In this example, RAG can help an organization's HR department streamline the process of ensuring that they’re keeping up with the latest advice by efficiently retrieving relevant legal documents.
Key considerations for RAG
If your organization has identified a use case, it's important to consider various implementation factors to ensure the effective deployment of a RAG workflow. Here are a few key questions to consider:
Do I need live data from multiple sources?
Integrating live data from multiple sources into an AI data pipeline for a RAG workflow offers several advantages. First, by incorporating data from multiple sources, RAG can provide a comprehensive view of the relevant information, enhancing the relevance of its generated outputs. This integration allows RAG to leverage a diverse range of data sources, such as emails, chats, and documents from various applications, enabling a more thorough analysis and synthesis of information. Second, live data ensures that the information used by RAG is always current, enabling access to the most up-to-date insights and facilitating a timely decision-making process.
However, there may be situations where integrating live data from multiple sources is not necessary. For instance, if the legal use case primarily involves static data that does not require frequent updates, such as historical legal documents or archived contracts, integrating live data may be unnecessary. Similarly, if the scope of the RAG workflow is limited to a single data source or collaboration application, there may be no need to incorporate data from multiple sources, especially if the application provider offers in-app RAG-enabled features.
Should I build or buy the infrastructure needed for RAG?
An enterprise might opt for an AI data pipeline solution that integrates and maintains the necessary tools for automating a RAG workflow for several reasons. First, such a pipeline provides a more streamlined and cohesive solution, eliminating the need for piecemeal integration and maintenance of individual tools. Additionally, an integrated AI data pipeline often includes dedicated support and maintenance services, ensuring reliability, scalability, and ongoing updates to meet evolving business needs. What’s more, a pre-built AI data pipeline may offer advanced features and customization options tailored to specific use cases, enhancing flexibility and efficiency in implementing RAG workflows.
However, there may be situations where building and maintaining different pieces of infrastructure or a set of open-source tools is preferred. Enterprises with ample in-house expertise and resources might choose to develop and maintain their tools to align more closely with their internal workflows and processes.
Ultimately, the decision to use an AI data pipeline or not depends on the specifics of the use case related to data sources and access, technical capabilities, and the strategic objectives of deploying RAG workflows.
Generative AI journey and building a data foundation
For legal professionals at any stage of their GenAI journey, be it the exploratory phase or the active consideration of an AI data pipeline for RAG, one fundamental remains consistent: investing in your data is an essential first step.
Investing in proprietary data management allows organizations to centralize and organize their data assets, making them more accessible and actionable for various purposes, including potential future AI implementations. A robust data foundation enables organizations to gain deeper insights into their operations, customers, and markets, facilitating informed decision-making and strategic planning.
In the context of legal and compliance, leveraging proprietary data can help organizations enhance their risk management protocols, compliance practices, and legal discovery processes by providing a comprehensive view of relevant information.
Even if an organization is not yet ready to implement GenAI applications, building a data foundation can automate an organization's mission-critical workflows while laying the groundwork for future initiatives, ensuring that they have the necessary infrastructure and resources to leverage AI technologies responsibly and effectively when the time comes.
Interested in leveraging your proprietary data with Generative AI and seeing a RAG workflow in action? Reach out to learn more about Onna’s AI Data Pipeline beta, which enables you to use your data with LLMs securely. For more information, visit our solution page or get in touch here.