The IT Manager’s guide to measuring the impact of data management: KPIs and metrics to use
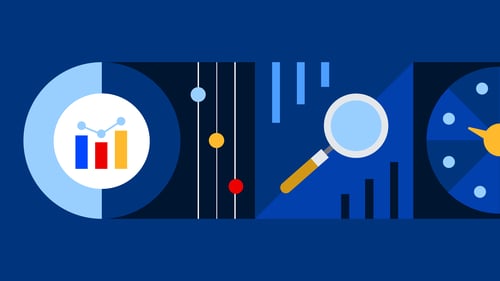
Introduction
As regulations tighten and interest in AI and ML technologies grows, effective data management has become indispensable for organizations. This encompasses data governance, records retention, and prevention of spoliation, enabling businesses to stay compliant, secure, and competitive.
As an IT Manager, you hold a critical position in managing your enterprise's data, ensuring its accuracy, availability, security, and compliance. But while you focus on avoiding incidents and extracting valuable insights from data, it's equally important to assess the effectiveness of your data governance initiatives. To communicate the value of a data management program to the C-suite, you’ll need subjective measures plus the numbers to back it up.
Here’s why it’s so important to know how effective your data management program is, followed by a series of key metrics — both qualitative and quantitative — that can help you gauge its impact.
Why analyze your data management program?
Measuring the success of data management initiatives is essential for many reasons, and using key performance indicators (KPIs) provides a way to evaluate the efficacy of your data-related strategies and processes. You can’t just set a strategy and forget it. Failing to establish a measurement plan will make it easier for data governance to fall by the wayside as other measurable initiatives take priority.
KPIs are crucial as they deliver insights to executives, helping them make informed choices about investments, strategy adjustments, and resource allocations that align with overall business goals. These goals may include:
- Improving regulatory compliance
- Reducing data storage costs
- Lessening the administrative burden on IT staff
- Mitigating litigation risks
- Increasing productivity
- Securing confidential information
Without metrics, you won’t know whether your organization is moving in the right direction to achieve its goals or be able to demonstrate the value of your program to stakeholders.
You can also use KPIs to determine areas that need improvement. Without data, you won’t know whether you need to adapt your processes to fall in line with industry standards and benchmarks. Data can also motivate greater engagement among business units, especially if they know they are falling behind competitors or even other internal departments. You can also demonstrate how seriously you take data security to customers and partners, encouraging them to do more business with your company.
Finally, KPIs can boost your organization’s productivity by helping you manage your data assets. You can learn which datasets are being used and which are being neglected and just costing you money to store. You can then archive or dispose of that data, freeing up resources, saving on costs, and making it easier to find higher-value data.
But what measures should you use? The following list provides a starting point for qualitative and quantitative measures that you can tailor and expand as you define what success looks like for your organization.
Qualitative signs of data management success
Qualitative indicators reflect the positive impact of data management efforts on an organization. While they might not be quantifiable, these signs provide valuable insights into the effectiveness of data management practices.
Subjective signs of data management success include the following:
- Improved decision-making: Accurate and reliable data, readily available for analysis, empowers organizations to make informed, strategic choices that drive business growth.
- Enhanced operational efficiency: Efficient data management streamlines workflows, reducing the time and effort required to access, analyze, and use data. Operational efficiency translates into increased productivity, allowing employees to focus on value-added tasks instead of grappling with data-related challenges.
- Stronger compliance: Organizations that effectively manage their data are better equipped to adhere to legal and regulatory requirements and avoid fines, penalties, and reputational damage.
- Cost savings: By reducing unnecessary data storage costs, streamlining processes, and minimizing legal risks associated with data mismanagement, organizations can optimize their budgets and allocate resources more efficiently, leading to sustainable growth and profitability.
- Reduction in litigation risks: Strict data management practices help preserve relevant data and eradicate irrelevant data defensibly.
- Cost reduction: Proper data governance cleans and organizes data, reducing redundancies and inconsistencies. Streamlined data can lead to significant cost savings in terms of storage, data processing, and personnel hours.
- Innovation and agility: A proactive data management approach fosters organizational agility and innovation, facilitating quick adaptation to market changes and opportunities.
- Risk management and resilience: Enhanced data management practices contribute to better risk identification and mitigation, improving organizational resilience against operational challenges.
- Brand reputation and trust: Demonstrating a commitment to data privacy and security through effective data management can enhance a company's reputation and build trust among stakeholders.
- Strategic alignment and execution: Aligning data management practices with business objectives ensures that strategic initiatives are supported and executed effectively, driving overall business success.
Quantitative data management metrics
Quantifying the impact of data governance can be challenging due to the multifaceted and often intangible nature of its benefits, but organizations can rely on several KPIs to assess the effectiveness of their data governance efforts. These metrics provide insights into various aspects of data quality, compliance, security, and business value.
Here are some metrics and KPIs that can be used to evaluate data governance tailored to different categories of data management goals.
Data quality metrics
Data quality refers to how accurate, reliable, complete, and relevant data is for its intended use in a specific context. High-quality data is essential for making informed decisions, conducting accurate analyses, and ensuring the success of various business processes. In contrast, poor data quality has the potential to destroy business value. Gartner research has found that organizations believe poor data quality is responsible for an average of $15 million per year in losses.
Increasing data quality involves multiple steps, including data cleansing (identifying and correcting errors or inconsistencies), validation (verifying data against predefined rules), and verification (validating data accuracy through cross-referencing and other checks). Data quality management often involves implementing data governance policies, data profiling tools, and data validation protocols to maintain and improve data quality over time.
Here are some data quality metrics to measure:
- Accuracy: To measure data accuracy, compare the data to a trusted source or conduct periodic data audits. How many errors does your data contain? Does it represent the real-world values that it’s supposed to depict?
- Completeness: Missing or incomplete data can hinder analysis and result in incomplete or biased results. Does your data contain all the necessary information required for its intended purpose? Are all required data fields populated and complete?
- Consistency: Inconsistent data, such as data that uses different units of measurement or date formats, can lead to confusion and misinterpretation. Does your data follow the same format and standards across different databases and systems?
- Timeliness: Timely data is up-to-date and relevant for the current analysis or decision-making process, while outdated data can lead to misinterpretations because it may not reflect the current state of your business. How quickly is your data captured? How quickly is it processed and made available? How old is your data?
- Relevance: Including irrelevant data in analyses can add noise and reduce the accuracy of results. How relevant is your data to the task at hand?
- Validity: Invalid data — data that does not meet the rules or constraints of a data set — can lead to erroneous interpretations. How well does your data adhere to defined rules?
- Integrity: Data integrity ensures that the relationships between different data elements are maintained. How many inconsistencies arise in data storage, processing, and transmission?
Compliance and security metrics
These KPIs indicate how well organizations adhere to regulatory requirements, industry standards, and internal policies related to data protection and security. They help organizations evaluate their compliance posture and the effectiveness of their security measures.
Here are some examples of compliance metrics:
- Compliance status: How well does your data adhere to data protection regulations (e.g., the General Data Protection Regulation (GDPR), the California Consumer Protection Act (CCPA), and the Health Information Portability and Accountability Act (HIPAA))? Does it follow your internal data policies?
- Policy adherence: How well do employees comply with internal data security policies and procedures? How many mistakes do they make?
- Audit findings: How many data-related problems came to light during internal or external audits? How costly and time-consuming were these issues to resolve?
- Incident response time: How long does it take to respond to data security incidents or breaches?
- Training completion rates: How many employees have completed mandatory compliance training programs, and how frequently are these trainings conducted?
- Data classification accuracy: How well do your systems categorize data? How well do you capture and protect confidential, proprietary, personal, or otherwise sensitive data?
- Vendor compliance: How well do third-party vendors and partners handle your data? Are their data management practices aligned with your company’s standards and regulatory requirements?
And here are some suggested data security metrics. Regular monitoring and analysis of these metrics are crucial for maintaining a robust and effective cybersecurity program.
- Number of security incidents: How many security incidents (e.g., breaches and unauthorized access attempts) occurred over a specific period?
- Incident severity level: How severe were the security incidents? What were the threats to the business?
- Time to detect: How long did it take to detect security incidents?
- Time to contain: How long did it take to contain and mitigate the impact of security incidents after detection?
- Phishing click rates: How many employees click on phishing links in simulated phishing exercises?
- Patch management effectiveness: How quickly are security patches applied to systems and applications after their release?
- User access reviews: How frequently do you review user access rights to ensure they align with the principle of least privilege (a user should only have access to the data needed to complete a task)?
- Encryption usage: What percentage of sensitive data is encrypted, both at rest and in transit?
- Intrusion detection/prevention system alerts: How many alerts are generated by these systems to identify potential security threats? How many threats are missed?
- Firewall rule reviews: How well do firewall rules prevent unauthorized data access?
Business value metrics
In the context of data governance, business value metrics refer to KPIs that measure the impact and effectiveness of data management initiatives on overall business objectives and outcomes. These metrics help organizations assess the ROI of their data governance practices, enabling them to make informed decisions to improve data-related processes.
Here are some examples of business value metrics:
- Data quality improvement: How much do data accuracy, completeness, and consistency improve after implementing data governance practices?
- Compliance adherence: How well does the organization comply with laws and regulations (such as GDPR and HIPAA)? How well does it comply with internal data governance policies? How many fines or penalties have been assessed against the company?
- Data accessibility: How quickly and easily can users access required data?
- Data usage metrics: How often do departments or teams use data to make decisions?
- Data-driven innovation: How many new products, services, or business strategies are developed using insights derived from governed data?
- Data security effectiveness: How many data security incidents occur over time? How many data breaches do your security measures prevent?
- Cost reduction: How much money do you save with reduced data storage? With improved data utilization?
- Business process optimization: How efficient are your business processes as a result of improved data governance? How much time do your people save in searching for the information they need?
- Customer trust and satisfaction: How do customers perceive your company based on your data privacy and security measures? What are the changes in customer trust and satisfaction levels over time?
- Data monetization: How well does your organization generate revenue from data-based products or services?
- Data collaboration: How well do departments and external partners use data to collaborate?
- Data lifecycle management: How efficient are the organization’s data lifecycle management processes? How costly is data storage? What are your data disposal costs?
- Data governance maturity: How mature are your data governance practices? How have they evolved over time?
Operational efficiency metrics
Operational efficiency metrics focus on evaluating the effectiveness and productivity of data-related processes and activities within an organization. These metrics help assess how well data governance practices contribute to streamlined operations, reduced manual efforts, and optimized resource utilization.
Here are some examples of operational efficiency metrics:
- Data processing time: How long does it take to transform raw data into a usable format for analysis, reporting, or other business activities?
- Data retrieval time: How long does it take to retrieve records or datasets from storage systems?
- Data entry error rates: How many errors occur in manual data entry processes?
- Data integration efficiency: How quickly and easily can you integrate and harmonize data from various sources for analysis?
- Data duplication rates: How many duplicate data records exist in your databases and systems?
- Data cleanup time: How long does it take to identify and correct data inconsistencies, errors, or redundancies?
- Data governance policy compliance: How well do employees and systems adhere to data governance standards?
- Automated data validation: How many data validation tasks are automated? How many remain manual? How long do those manual processes take?
- Data access permissions: How long does it take to grant or revoke data access permissions for employees and systems?
- Data cataloging time: How long does it take to catalog new datasets?
- Data lifecycle management efficiency: How long does it take to archive data? To purge old data?
- Query performance: How quickly can you execute database queries?
- Data backup and recovery time: How long does it take to back up data and restore it in the event of data loss?
- Data governance training: How often do you train employees on data governance policies and best practices? How prevalent is non-compliance among the trained employees?
Data monetization metrics
Data monetization metrics evaluate the financial value generated from data assets. They assess the effectiveness of data governance practices in enabling organizations to capitalize on their data assets.
Here are some examples of data monetization metrics, though it's important to note that the relevance and applicability of these metrics can vary significantly depending on the specific company and industry context. Organizations should select and tailor these metrics to align with their unique business objectives and market dynamics:
- Revenue from data products: What is the total revenue generated from products or services directly based on data, such as data analytics reports, market insights, or predictive models?
- Customer segmentation revenue: How much revenue stems from targeted marketing campaigns enabled by data-driven customer segmentation?
- Personalization revenue: How much revenue results from creating personalized customer experiences and product recommendations?
- Data subscription revenue: How much revenue do you generate from subscription services where customers pay for access to specific datasets or premium data insights?
- Data licensing revenue: How much revenue do you earn from licensing data to external organizations, partners, or researchers for their analyses or applications?
- Cross-selling and upselling revenue: How much do you earn from cross-selling and upselling efforts directed toward existing customers that are facilitated by data-driven insights?
- Operational efficiency savings: How much money do you save by optimizing internal processes, supply chain management, or resource allocation through data-enabled insights?
- Fraud detection and prevention savings: How much do you save by preventing fraudulent activities, including reduced financial losses and mitigation of legal costs?
- Customer retention savings: How much do you save by retaining existing customers through data-driven retention strategies? What is the impact on your customer acquisition cost?
- Inventory optimization savings: How much do you save on optimized inventory management based on accurate, data-driven demand forecasts?
- Dynamic pricing revenue: How much revenue results from dynamic pricing strategies adjusted in real-time based on market demand and customer behavior data?
- Predictive maintenance savings: How much can you save from reduced equipment downtime and maintenance costs achieved by implementing predictive maintenance models?
- Advertising revenue: Measures revenue generated from targeted advertising efforts facilitated by data governance practices, ensuring ads are shown to the right audience.
- Customer lifetime value (CLV) increase: Calculates the increase in CLV resulting from personalized customer experiences and loyalty programs driven by data insights.
- Brand value enhancement: Measures the enhancement in brand value resulting from data-driven marketing campaigns, improved customer satisfaction, and positive customer interactions.
Risk management metrics
Risk management metrics assess how well organizations mitigate data-related risks. These KPIs help organizations identify, evaluate, and manage potential risks to data security, privacy, compliance, and overall integrity.
Here are some examples of risk management metrics:
- Data breach incidents: How many data breaches or incidents involve unauthorized access, disclosure, or loss of sensitive data?
- Incident response time: How long does it take to detect, assess, and respond to data security incidents or breaches?
- Data security compliance: How well does the organization comply with data security standards and regulations, such as GDPR, CCPA, and HIPAA?
- Sensitive data exposure: How often is sensitive or confidential data exposed to unauthorized users or external entities?
- Data encryption usage: How much data is encrypted at rest and in transit?
- Access control effectiveness: How effective are access controls and user permissions?
- Data loss prevention effectiveness: How effective are data loss prevention solutions in preventing the unauthorized transfer or disclosure of sensitive data?
- Compliance audit findings: What are the findings and recommendations from internal and external data governance and security audits?
- Vendor and third-party risk: How well do third-party vendors and partners secure data? How many third-party data breaches or incidents occur?
- Data retention policy compliance: How well does the organization follow records retention policies? How much data is kept past its retention schedule?
- Training and awareness: How often does the organization train employees on data security? How many people attend the training?
- Incident severity levels: How many data-related incidents occur, and how severe are they? What response do they require?
- Data integrity: How accurate is your data? How reliable is it? How much data has been tampered with or altered?
- Regulatory fines and penalties: How many fines and penalties have been assessed against the organization for noncompliance with data protection laws and regulations? What is the cost of these fines and penalties?
- Risk assessment scores: What is the risk score for the data assets in your organization? How well do you assign security measures to the riskiest assets?
Take a proactive approach to measuring your data governance initiatives
Data governance isn’t a set-it-and-forget-it strategy; it’s an ongoing process that demands careful oversight and iterative improvement to stay effective. At a time when budgets are under heavy scrutiny, measuring the impact of data management initiatives is crucial for demonstrating their value to stakeholders and securing the necessary support and resources for their success.
Establishing and monitoring key performance indicators is essential for assessing effectiveness and aligning data management practices with organizational goals. This approach enables businesses to efficiently manage their data assets and enhance their value.
To maintain the success and relevance of your data management program, it’s advisable to continuously refine these metrics, adapt to technological advancements, and sustain a culture that values and understands the importance of strong data governance.
Learn how Onna's centralized platform can eliminate the headaches of governing your digital workplace data by visiting our Demo Center or getting in touch here.
Other posts you might be interested in
View all posts
Webinars
8 min read
Webinar recap: The new normal of data preservation
Read more
Guides
16 min read
The IT Manager’s guide to measuring the impact of data management: KPIs and metrics to use
Read more
Blogs
6 min read